Predictive maintenance describes the action of being able to detect potential problems at an early stage using comprehensive data analyses before they lead to a breakdown in productivity. Predictive maintenance thus protects against problems related to potential hazards to people and leads to an improvement in reliability, safety, availability, efficiency and quality. [1]
Predictive maintenance has been made possible by increased computing capacities and lower costs for sensors over the last decades. Sensors play a central role in predictive maintenance, as they make it possible to create a holistic digital picture of reality. In the past, correlations first had to be discovered and then specifically monitored. Since the cost of sensors has fallen, computing capacity has increased and more sensors have found their way into many areas, it is now possible to discover new correlations that were not previously investigated.
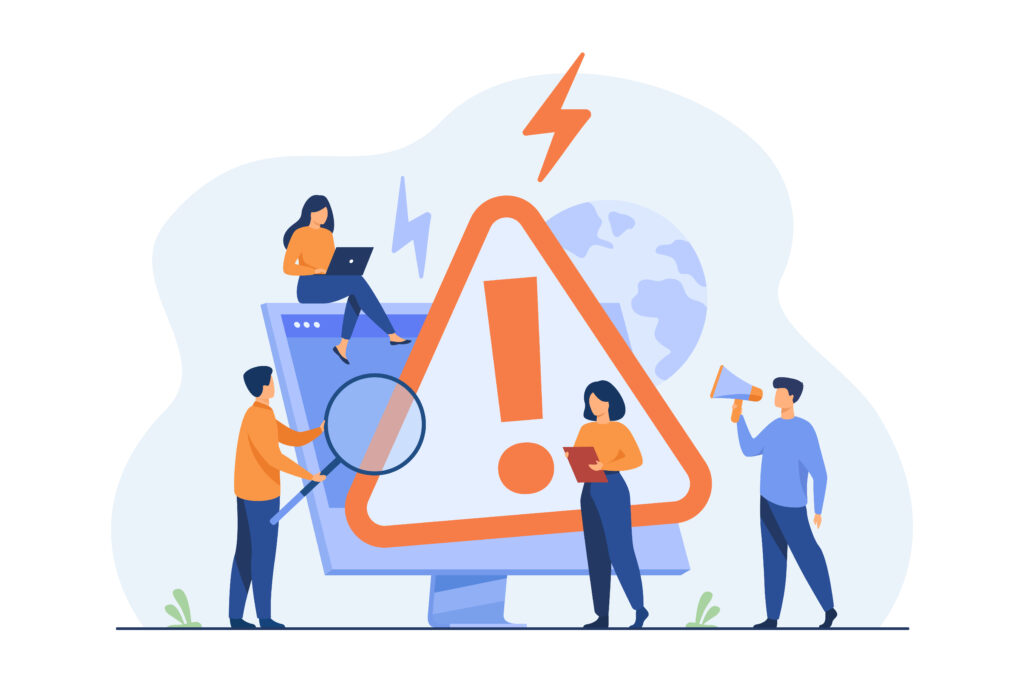
Challenges of Digital Twins
When implementing Digital Twins, there are some challenges that need to be addressed. These would otherwise also affect reliable predictive maintenance and other services relying of Digital Twins. The basis of a correct Digital Twin is to describe the actual state and not the target state. This means that, for example, not only CAD plans of a planned asset (machine, building, etc.) may be used to create the Digital Twin. During the realization of projects, it may be necessary to make spontaneous changes, e.g., to solve problems or to react to changed circumstances. However, these changes are often not incorporated into the previously created plans. As a result, the Digital Twin may not correspond to reality if it is based on plans that have been drawn up. [2]
Special attention must also be paid to maintenance work. If the system has been in operation for a long time and parts are replaced, these changes, no matter how small and marginal they may seem, must also be transferred to the Digital Twin to sustain a holistic view. [2]
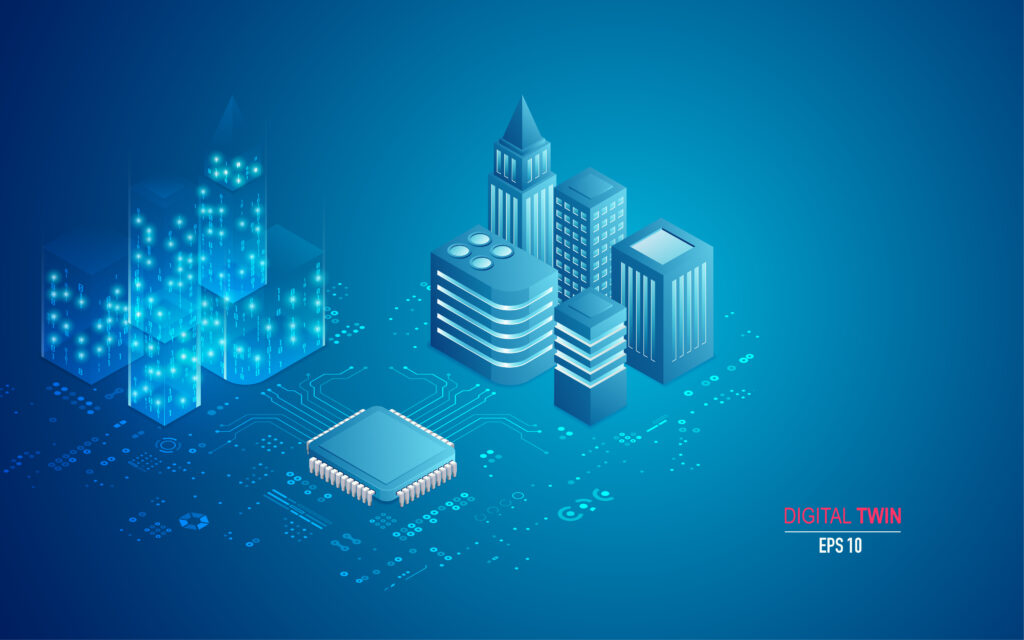
Predictive Maintenance and Digital Twins in Construction
As in many industrial sectors, predictive maintenance is also finding its way into the construction sector. The reason for this, in addition to the reduced cost of implementing sensor technology, is the high cost of breakdowns and problems. These include with labour costs, costs for spare parts, costs for consumables and delays in contracting and the possible loss of reputation a wide range of direct and indirect factors. [3]
The gradual introduction and adaptation of BIM paves the way for consistent digitalisation in the construction sector. It is therefore guaranteed that this step will not only increase the consistency of information from design to construction, but also the general quality. [4]
Predictive Maintenance takes the logical next step and continues the digitalisation through construction. Where BIM stops, Predictive Maintenance starts and delivers effective and efficient building management, especially during operation and maintenance (O&M). [5]
Through this combination of BIM and predictive maintenance, a holistic picture of the building is created with the help of Digital Twins to support the entire O&M process. This makes it possible to detect previously hidden correlations and to take care of work that arises before it turns into serious errors.
Tools To Pave the Way Towards Predictive Maintenance With Digital Twins
Currently there is no defined standard for tools. In particular, the definition of a standard for the exchange of data (modelling data, sensor data, etc.) must be prioritized in the next years. [6]
On the other hand, more and more tools are being developed to support O&M management in the best possible way. These include computerised maintenance management systems (CMMSs), computer-aided facility management (CAFM) systems, building automation systems (BASs) and integrated workplace management systems. [7]
In addition, there are many tools for the sub-areas of mapping digital twins. These differ greatly from the focused use case: Digital Twins of an entire city are significantly more complex than a local machine with firmly defined processes. [8] Therefore, there is no one possible toolset. To illustrate the complexity, the list by Qi et al. [8] provides an overview of the software areas that must interact in digital twins [8]:
- Tools for digital twin modelling (e.g., Tools for physical modelling, Tools for rule modelling, Tools for geometric modelling)
- Tools for connections in digital twin (e.g., Tools for connections between physical and digital spaces, Tools for connections within digital spaces)
- Tools for the physical world (e.g., Tools for sensing the physical world)
- Tools for digital twin data (e.g., Tools for data transmission, Tools for data storage, Tools for data visualization)
- Tools for digital twin services (e.g., Optimization service tools)
Examples of tools that cover several of these areas are: Predix, PTC’s Thingworx, Siemens’ MindSphere, ANSYS, Dassault’ 3D Experience and Foxconn’s Beacon [8]
Forecast
With ever-increasing computing capacities and ever-decreasing costs to integrate comprehensive sensors into real world assets, digitalisation in the building sector will continue to evolve and pave the way towards digital twins. This then offers the possibility to map reality almost identically and thus to potentiate operation and maintenance management (O&M) with the help of predictive maintenance.
In the end, the goals are clear: reduced failure times, increased productivity and finally – lower costs. Standards, fundamentals, and toolsets still need to be defined along the way. [9] Then predictive maintenance will bring the desired success.
Written by Tobias Hinz, from Ascora
[1] Selcuk, S. (2016). Predictive maintenance, its implementation and latest trends. Proceedings of the Institution of Mechanical Engineers, Part B: Journal of Engineering Manufacture, 231(9), 1670–1679. https://doi.org/10.1177/0954405415601640
[2] Pfenning, M. (2021). Per Digital Twin zur erfolgreichen Predictive Maintenance. Instandhaltung, Moderne Industrie, Lansberg, Germany. See https://www.instandhaltung.de/instandhaltung-4-0/per-digital-twin-zur-erfolgreichen-predictive-maintenance-205.html (Accessed 22/04/2021)
[3] Edwards, D. J., Holt, G. D., & Harris, F. C. (1998). Predictive maintenance techniques and their relevance to construction plant. Journal of Quality in Maintenance Engineering, 4(1), 25–37. https://doi.org/10.1108/13552519810369057
[4] Chen, L., & Luo, H. (2014). A BIM-based construction quality management model and its applications. Automation in Construction, 46, 64–73. https://doi.org/10.1016/j.autcon.2014.05.009
[5] Lu, Q., Xie, X., Parlikad, A. K., Schooling, J. M., & Konstantinou, E. (2020). Moving from Building Information Models to Digital Twins for Operation and Maintenance. Proceedings of the Institution of Civil Engineers – Smart Infrastructure and Construction, 1–9. https://doi.org/10.1680/jsmic.19.00011
[6] Aivaliotis, P., Georgoulias, K., & Alexopoulos, K. (2019, June). Using digital twin for maintenance applications in manufacturing: State of the Art and Gap analysis. 2019 IEEE International Conference on Engineering, Technology and Innovation (ICE/ITMC). 2019 IEEE International Conference on Engineering, Technology and Innovation (ICE/ITMC). https://doi.org/10.1109/ice.2019.8792613
[7] Sapp, D. (2017). Whole Building Design Guide. National Institute of Building Sciences, Washington, DC, USA. See http://www.wbdg.org/om/om.php (Accessed 15/04/2021)
[8] Qi, Q., et al. (2019). Enabling technologies and tools for digital twin. Journal of Manufacturing Systems. https://doi.org/10.1016/j.jmsy.2019.10.001
[9] Aremu, O. O., Palau, A. S., Parlikad, A. K., Hyland-Wood, D., & McAree, P. R. (2018). Structuring Data for Intelligent Predictive Maintenance in Asset Management. IFAC-PapersOnLine, 51(11), 514–519. https://doi.org/10.1016/j.ifacol.2018.08.370